How should government and business manage risks emerging from AI systems?
MPP student Bálint Pataki sets out the strategies needed to manage risks from AI
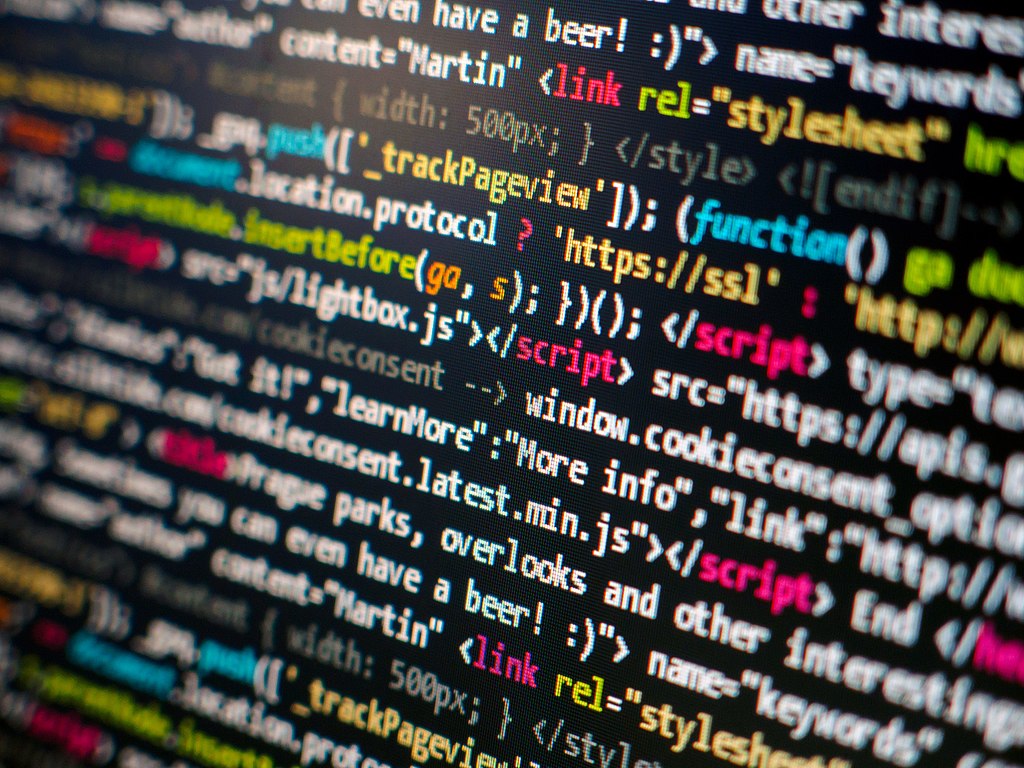
Artificial Intelligence (AI) is an umbrella term for computer programs that have human-like capabilities such as language, visual perception and motor control. State-of-the-art AI systems are often praised for the benefits they may bring to the many challenges facing our societies - for example faster scientific progress, or the potential to enable a more sustainable future – but how can governments and business reap the benefits of AI while minimising its damaging effects?
What are the potential options for corporate and governmental AI risk management, and how can businesses and policymakers implement them to make the development and deployment of AI more trustworthy i.e. lawful, ethical, and technically robust, without necessarily limiting the creation of societal benefits or the potential profits of innovation?
Mapping out the risks
Business and government need to understand the kinds of risks AI poses to enable them to create adequate management strategies.
For example, bias and discrimination risks, where ethnic, religious and other forms of algorithmic bias can lead to discrimination, could be dealt with through trialling AI systems with stakeholders to detect biases.
Psychological harm and emotional harm is another risk: the death of a teenager in the UK was attributed to Instagram’s algorithm. Risk management strategies here might include a greater use of psychologists in the development and deployment of AI systems.
And how do we know that what we read, see or hear is true? Berlin’s Mayor spoke for 15 minutes with a deepfake (AI video) version of Vitali Klitschko before suspecting fraud. Ensuring that all systems creating convincing content have watermarks showing that the content was AI generated is an option to manage these risks.
Alongside the very real privacy fears arising from mass surveillance, and the potential for significant environment damage - training an AI system can emit more than 250000 tons of CO2 - AI risk management requires sound implementation frameworks.
Governments can mandate or facilitate, and businesses can independently use the following three implementation strategies to address the above risks:
- Risk management by design: From development to deployment and use, risks must be understood and effectively mitigated across the AI lifecycle. This already starts at the ideation stage where companies developing and deploying AI should ask themselves “What could go wrong?”. Depending on how the AI model is being used, risk management responsibility continues, for example, through monitoring misuse even after finishing the development and deployment of an AI system.
- Internal and third-party audits: Especially for AI models that are used in a variety of applications, audits can significantly contribute to trustworthiness. It can help ensure that AI models are designed and deployed in ways that are ethical, legal, and technically robust. Such audits can either be performed by internal teams or by third parties to capture the risks posed by AI.
- Reporting risk factors and incidents: Industry, governments and the wider society can better imagine and act upon AI risks if risk factors and potential bad outcomes are known. A way to inform society of bad outcomes is by reporting near-misses, i.e., events that nearly ended up bringing about bad outcomes. However, actors would likely only report near-misses when they know that they will not be blamed for nearly causing issues. Individual corporate governance practices can thus contribute to a more trustworthy AI ecosystem.
So what are the next steps for leading AI developers and policymakers?
Leading AI-developing firms could:
- adopt risk management practices throughout their value chains, in anticipation of demands from customers and governments;
- join together with other leading developers for a Code of Conduct across the industry to disseminate state-of-the-art trustworthiness practices; and
- adopt higher trustworthiness standards than strictly demanded externally. Internalising voluntary AI risk frameworks, such as the US government’s recommended NIST guidelines, could signal commitment to be a responsible, social-minded and ethically trailblazing companies.
Policymakers could:
- establish minimum requirements for AI risk management across the developers and deployers of AI systems through legislation, similar to the upcoming EU AI Act;
- set up AI audit capacities to ensure compliance related to risk management, investigate specific incidents and to assess the overall risk exposure of companies; and
- incorporate AI risk management into public procurement practices to avoid creating a market for AI systems posing unacceptable risks.
Developing and deploying AI systems is a huge opportunity for productivity gains and scientific progress. However, the dangers that this progress holds also necessitate that companies and governments seriously address their novel risk management responsibilities.
I am thankful for the valuable contributions and insights from Sabrina Küspert, Dr Roxana Radu and Dr Keegan McBride.